Dr. Angela Di Fulvio
Associate Professor at the University of Illinois Urbana-Champaign
Lectures
Detectors and Algorithms for Neutron Spectroscopy
Neutrons are ideal probes for examining nearly all forms of matter. However, their distinct properties make their direct spectroscopic characterization a challenging task. Traditional deconvolution-based spectroscopy methods require prior knowledge of the neutron field and yield neutron spectra with coarse energy resolution.
Deuterium-based scintillators can measure neutron energy spectra efficiently by suitably unfolding the neutron light-output spectra. The non-isotropic scattering reaction between interacting neutrons and deuterium produces distinct edges in response to monoenergetic neutrons that facilitate spectrum unfolding and make deuterated scintillators particularly suitable for high-resolution neutron spectroscopy.
I will present the light output response of a new deuterated stilbene crystal to neutrons and alpha particles and related light-quenching parameters and its excellent pulse shape discrimination capability. I will also introduce the use of statistical inference methods to solve the inverse unfolding problem, inherently providing the a-posteriori uncertainty associated with the spectrum. I will present experimental results that show the ability of deuterated stilbene and data analytics to accurately characterize and discriminate the neutron spectra emitted by various sources, including fission from highly enriched uranium and weapons-grade plutonium and fusion from deuterium-deuterium and deuterium-tritium reactions.
Fuel Accountability and Monitoring at Advanced Nuclear Reactors
Realizing the market potential for advanced reactors across the wide breadth of proposed end-use applications will require a paradigm shift in fuel accountability and reactor monitoring and control.
In this talk, I will present new concepts for reactor monitoring and accountability of nuclear fuel, with particular emphasis on tristructural-isotropic (TRISO) pebbles, as employed in pebble-bed reactors (PBRs) and modular high-temperature gas reactors. These non-destructive examination (NDE) methods can improve fuel accountability and management during reactor operation and at the back end of the fuel cycle.
Two nondestructive assay (NDA) methods for TRISO-fueled pebble characterization, namely neutron multiplicity counting and X-ray computed tomography, will be presented. Based on these two NDE methods, a unique pebble identification can be achieved in a few minutes to meet the operational constraints of PBRs.
Machine Learning for Radiometric Clustering and Classification
Machine learning algorithms’ capability to perform non-linear classifications and clustering is particularly valuable in handling radiometric datasets, often including interdependent and complex signatures.
During this presentation, I will illustrate the practical application of machine learning algorithms in two distinct areas: pulse shape discrimination and image segmentation. Despite the apparent differences between these tasks and the different data domains, they share common challenges, namely solving clustering and classification problems on large data sets.
The pulse shape discrimination results show that supervised classifiers such as k-nearest neighbor, support vector machine, random forest, and variational autoencoders outperform traditional deterministic charge integration methods when discriminating gamma-ray and neutron pulses detected by organic scintillators. Notably, variational autoencoders necessitate minimal supervision and are adaptable across various radiation sources. Furthermore, I will discuss the need for advanced methods when dealing with three-dimensional data classification. Here, an adaptive point-cloud segmentation approach will be explored, aimed at reducing data size and computational time while ensuring precise segmentation. The method is successfully applied to segment the prostate in radiation therapy treatment planning, demonstrating its adaptability during successive treatment fractions by automatically adjusting to changes in organ dimensions and positions.
About
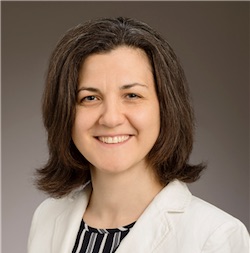
Dr. Angela Di Fulvio is an Associate Professor in the Nuclear Plasma and Radiological Engineering (NPRE) Department at the University of Illinois Urbana-Champaign (UIUC) and the director of the Nuclear Measurement Laboratory (NML). Prior to joining NPRE in 2018 as an Assistant Professor, she was a researcher at Yale University and the University of Michigan, where her work focused on the development of cutting-edge neutron multiplicity counters and superheated emulsion-based detectors. She is an author of over 130 papers published in peer-reviewed international journals and conference proceedings. For her scholarly work, she received several awards, including the 2022 UIUC Dean’s Award for Excellence in Research.
Her group at UIUC focuses on the design, simulation, and characterization of neutron detection systems. The group also develops Bayesian and machine learning algorithms to maximize the information gleaned from radiation measurements. Through the design and deployment of fast-neutron spectrometers, multiplicity counters, and associated algorithms, her work has contributed to ensuring the safe and secure utilization of nuclear materials and facilities.
Dr. Di Fulvio actively participates in professional organizations such as IEEE-NPSS, where she has been a member since 2014. In 2023, she furthered her engagement by joining the IEEE-NPSS Radiation Instrumentation Steering Committee (RISC) and serving as co-chair of the NPSS chapters.